Data preparation
Data preparation: Techniques and solutions with Zoho DataPrep
Data scientists and analysts devote 80 percent of their time to gathering, cleaning, and prepping data, but they spend only a fraction of that time on actual business analysis and delivering insights.
Businesses collect and store data from multiple sources, but it tends to suffer from quality issues, such as duplicates, errors, and missing or inconsistent data. Separate datasets also often have various formats or schemas that must be reconciled when merged, so these datasets need to be curated for accurate analysis and decisionmaking. This is where data preparation comes in.
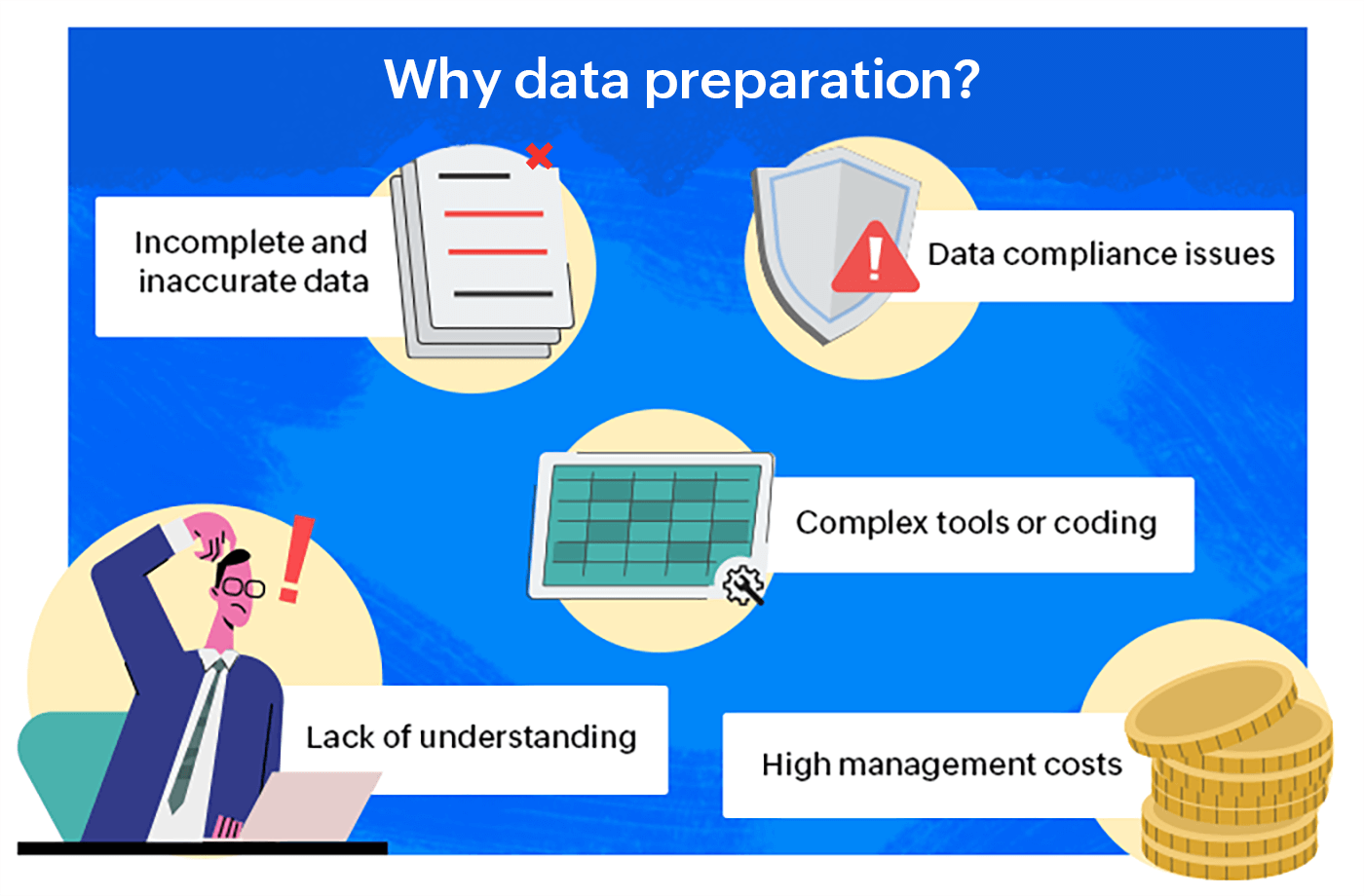
What is data preparation?
Data preparation is the process of collecting, combining, structuring, cleaning, transforming, and enriching raw data before warehousing, processing, and analysis. The prepared data can be used in business intelligence (BI), data visualization, and analytics tools, machine learning models, and more.
BI users surveyed by Dresner Advisory Services said that data preparation is one of the top technologies and initiatives for their BI deployments. These analysts rely on their IT departments to provide necessary business data—but not all organizations are equipped with an in-house IT department or data analyst to prepare data. This need is where self-service data preparation solutions came from.
What is self-service data preparation ?
Self-service data preparation is the process by which business users or individuals prepare and clean raw data for analysis without much technical knowledge or help from IT specialists. This method saves time and resources by allowing non-technical people to work directly with their data, which promotes faster decision-making.
The crucial role of quality data
Poor-quality data costs businesses an average of $15 million every year. The primary goal of data preparation is to organize and clean data so that it can be fed into data warehouses and business applications, preventing discrepancies.
In 2017, Uber had to repay its drivers “tens of millions” of dollars because of an accounting error that underpaid them for years.
In 2018, a “fat-finger” error, mistaking Korean won for shares by a Samsung Securities employee, cost the company $105 billion.
Both losses could have been avoided with relatively simple data processing techniques and systematic data validation. With proper data quality assurance methods, the company would have noticed the inaccurate formula computing its commission.
Quality data is crucial for many reasons, across different domains. Let's look at some other examples:
- In healthcare, accurate and reliable patient data is essential for effective diagnosis and treatment. Incorrect or incomplete data can lead to misdiagnosis and wrong prescriptions. Companies can also face lawsuits, penalties, and even lose their licenses to operate.
- Quality data is vital for personalized customer experiences. Ecommerce platforms rely on accurate customer data to recommend products, provide relevant discounts, and enhance overall user satisfaction. Inaccurate data can result in irrelevant recommendations and decreased customer trust.
- Marketing campaigns rely heavily on customer data to target the right audience. Poor data quality can result in ineffective targeting, leading to wasted resources and reduced campaign success.
- A simple mistake, such as sending promotional emails to unsubscribed users, can result in backlash and fines.
Benefits of data preparation
A major advantage of an efficient data preparation process is the ability of data professionals to concentrate more on data mining and data analysis—the parts of their work that create business value.
When data preparation is done right, it benefits businesses in many ways:
- Helps identify and fix errors quickly
- Ensures reliable data
- Eliminates duplicate work
- Facilitates better data-driven business decisions
- Frees more time for accurate insights
- Streamlines collaboration
- Improves data administration
Steps for preparing data
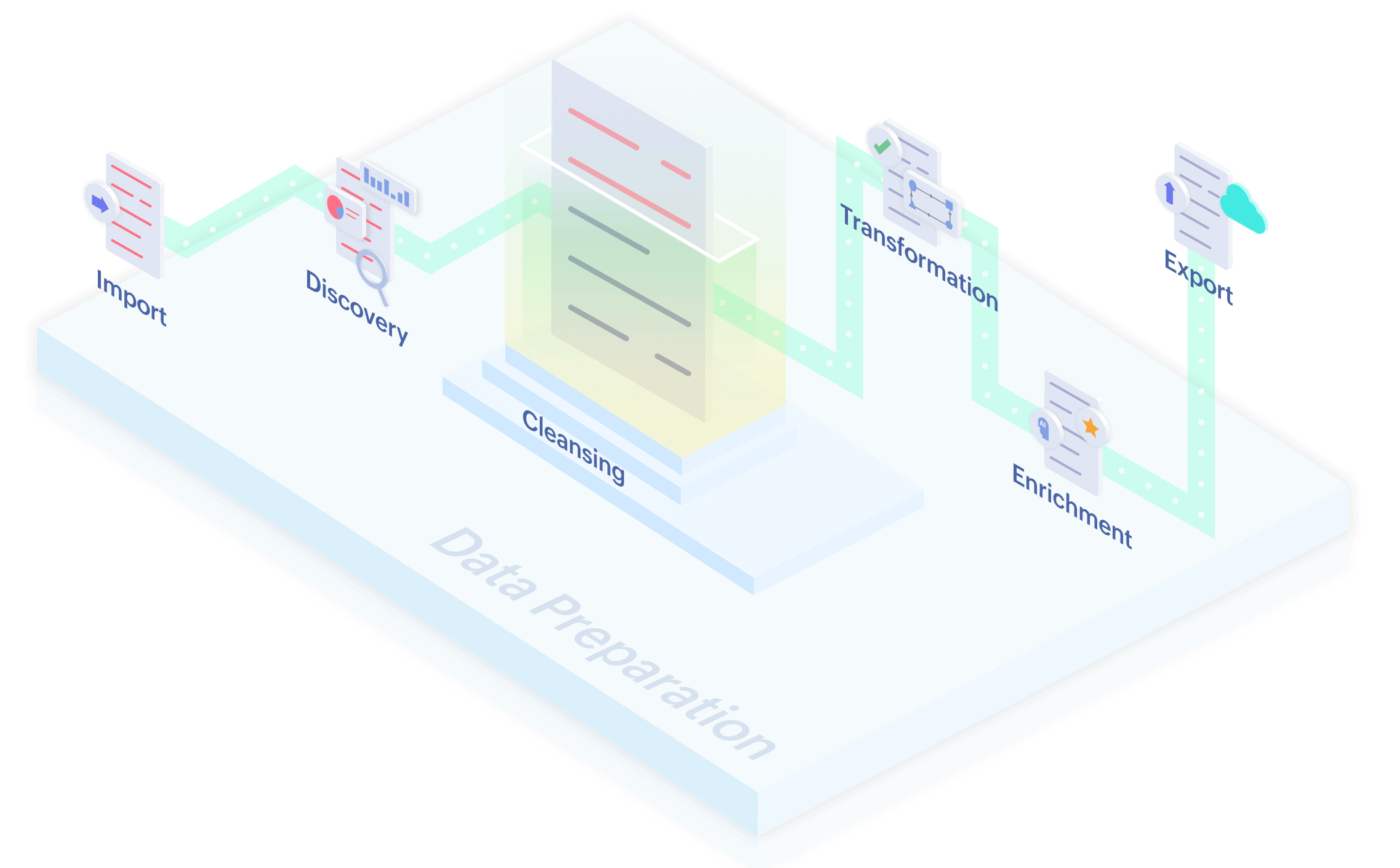
"There’s often a long up-front process of data collection, data cleaning, and data linking before the team can start to generate insights and impact for the client.
Data preparation involves a series of steps:
- Collection: Connect to multiple data sources and collect all relevant data.
- Modeling: Explore the collected data and perform data profiling by identifying patterns, anomalies, missing values, and other issues that need to be fixed.
- Cleansing: Improve data quality by eliminating identified errors, removing duplicates, and reducing inconsistencies.
- Structuring: Model and organize the data to a particular structure, to meet analytics requirements.
- Transformation: Transform data to your desired format.
- Enrichment: Enrich data with machine learning or third-party data.
- Validation: Set custom formats and data types, evaluate data quality and apply target matching.
- Pipeline: Create automated data preparation workflows.
- Management: Catalog data and manage data assets.
- Sharing: Set up secure data sharing and user groups to encourage collaboration.
Challenges in data preparation
Datasets compiled from many sources often have data quality, accuracy, and consistency issues. This messy data usually needs to be weeded out manually, which is tedious and time-consuming—in fact, 57 percent of data professionals feel that data preparation is their least enjoyable task, given its complexity. If preparing data requires a significant amount of work before it can be analyzed, taking action based on insights becomes more of a challenge.
Common data preparation challenges
- Collection of data from various sources
- Complexity involved
- Storage and accessibility of huge volumes of prepared data
- Lack of technical knowledge or human resources to prepare data
- Compliance issues
- Difficulty handling sensitive data
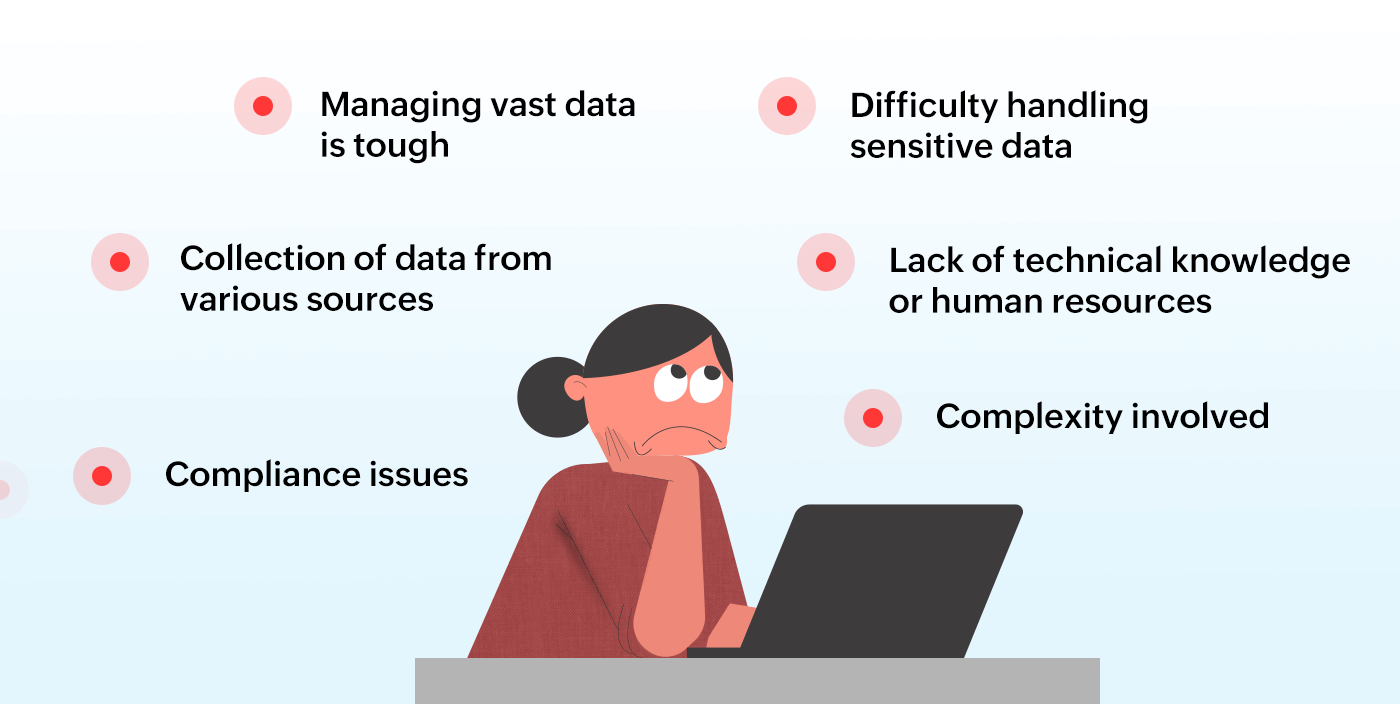
Data preparation solutions were previously reserved for large organizations due to resource constraints, complicated data preparation software needs, data security issues, scalability requirements, and big data collection complexity. But thanks to advancements in cloud computing and the democratization of data tools, even smaller firms can now easily use data preparation tools to simplify the data cleaning process and significantly cut data preparation time.
How Zoho DataPrep simplifies data preparation
It can take weeks of painstaking work before data is in a fit state for analysis to begin. But while data preparation is considered a tedious task, it can be made simple with Zoho DataPrep, an augmented self-service, cloud-based data preparation tool that helps to connect, transform, enrich, and clean data.
Here are a few ways Zoho DataPrep makes data preparation simple:
- Connect to 50+ data sources, including files, feeds, cloud storage, databases, warehouses, and business applications.
- Choose from auto-suggested transformations based on your data and perform smart cleanup using intelligent suggestions.
- Zoho DataPrep offers features powered by OpenAI, like transform by example, chat formula builder, and external dataset finder, and AI-based enrichment, like sentiment analysis, language detection, and keyword extraction, plus over 250 other transformations to enrich your data.
- Automating mundane tasks is one of the quickest ways to reduce data preparation time, and DataPrep allows you to schedule data preparation workflows and receive alerts.
- Data cataloging helps with data management and data discovery, based on the usage of data assets, their status, and associated meta information.
- Fine-grained permissions, privacy management, and secure handling of PII and ePHI data is crucial. With DataPrep, you can securely share data across your organization.
Zoho DataPrep can be used by professionals in data science, data analytics, and data engineering for business intelligence that empowers crucial data-driven decisions. Zoho DataPrep helps data scientists with data cleaning at scale, without any coding, to improve the effectiveness of machine learning models.
41% of marketers spend at least half of their time preparing data for use in campaigns and analysis. With DataPrep, businesses can avoid the pitfalls, minimize the costs, and quickly obtain high-quality data to gain accurate insights into their leads and prospects.